Exploring the Intersection of AI and Psychedelics: DMT Insights
Written on
Chapter 1: The Growing Interest in Psychedelics
In today's data-driven world, the decriminalization and medical application of psychedelics, including cannabis, are gaining traction. An exchange-traded fund (ETF) has even emerged, targeting pharmaceutical companies involved with psilocybin and other psychedelics, showcasing their potential as a lucrative market.
DMT, or N,N-dimethyltryptamine, is a key ingredient in the traditional Ayahuasca brew utilized by South American shamans for healing purposes. Beyond its therapeutic applications and impact on mental well-being, DMT is known for inducing vivid visual hallucinations, often characterized by intricate geometric patterns and vibrant visual experiences. For further insights into the various types of hallucinations, check out the enlightening lecture from the Harvard Psychedelic Society below:
Chapter 2: Advances in AI and Psychedelic Research
Recently, researchers from Imperial College London and Université de Genève have developed a model employing two generative deep convolutional networks to replicate psychedelic hallucinations associated with DMT. This concept is not entirely novel; a similar attempt was made in 2015 by Google DeepDream. However, the current study delves deeper into serotonin disruption and its effects on conscious experience.
DMT's molecular structure closely resembles that of serotonin, leading to significant benefits, particularly in treating depression. Nonetheless, its "alien" nature in the brain disrupts visual processing, causing sensory inputs from other modalities to be perceived visually, a phenomenon known as induced synesthesia.
The intricate geometric visuals experienced during a DMT trip stem from the disruption of standard visual processes. This includes the overlaying of persistent images, excessive application of 3D pattern fillers (similar to effects seen in films like Inception and Doctor Strange), and challenges in distinguishing edges, resulting in objects merging together. Additionally, asynchronous saccades and synesthetic experiences contribute to this unique visual landscape.
Why explore these disruptions using artificial intelligence?
Deep learning technologies, particularly generative adversarial networks (GANs), have become ubiquitous. These networks not only classify images but also generate them. There is a burgeoning interest from neurotechnology firms in leveraging psychedelics for therapeutic use, building on centuries of shamanic wisdom. Strong scientific evidence supports their efficacy for severe depression, and understanding the brain's reactions during these profound treatments can enhance care for patients, even if hallucinations are seen as mere side effects.
Therefore, despite AI and psychedelics originating from distinct fields, they can be combined to optimize treatment strategies. To simplify, GANs operate by utilizing a discriminator— a neural network trained on thousands of images to identify specific features (e.g., detecting cats in photos). Simultaneously, a generator creates synthetic images from random inputs until the discriminator can no longer tell the difference between real and generated images. This model has gained popularity, leading to the viral spread of deepfakes and even data augmentation in the medical sector.
A notable application of GANs is "style transfer," where the content of an image remains intact while adopting the stylistic elements of another image. For instance, envisioning your photograph as if painted by Van Gogh. This study's goal is to perturb a "traditional" image as perceived by the brain and transfer the "DMT style" onto it. Thus, GANs effectively recreate the synesthetic effects induced by DMT's brain alterations.
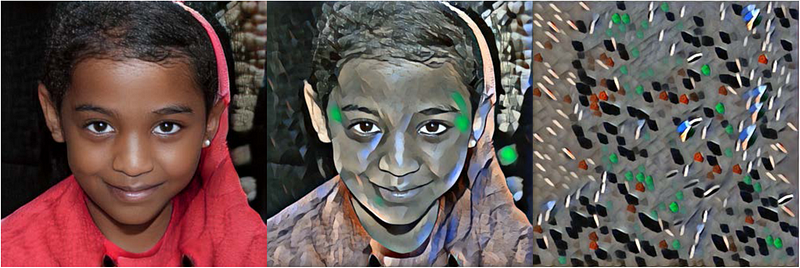
The architecture employed is the StyleGAN developed by Karras et al., as illustrated in the following figure.
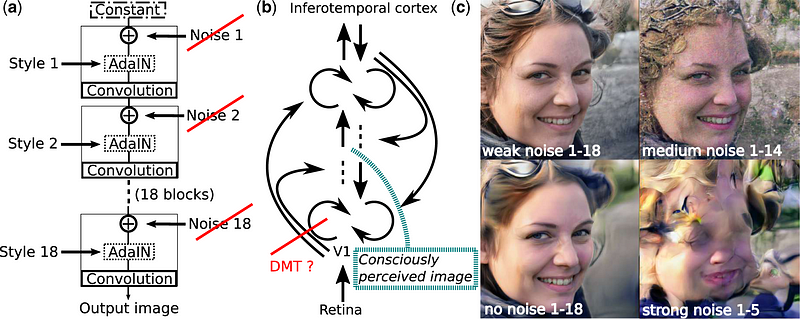
The human visual processing stream is widely regarded as crucial for visual experiences, making this research particularly promising as a computational method to replicate DMT-induced brain disturbances.
While this approach is not comprehensive enough to encapsulate the entirety of DMT and other psychedelics' effects—especially regarding serotonin receptor interactions—it represents a significant step forward. Our understanding of consciousness and perception remains limited, but this marks the beginning of a deeper computational representation of DMT experiences.
Chapter 3: Noteworthy Studies and Findings
References
- Schartnert Timmerman “Neural network models for DMT-induced visual hallucinations” Neuroscience of Consciousness 2021
- Karras, Laine, and Aila “A style-based generator architecture for generative adversarial networks”. In: Proceedings of the IEEE Conference on Computer Vision and Pattern Recognition. 2019, 4401–10.
- Davis, Alan K., et al. “5-methoxy-N, N-dimethyltryptamine (5-MeO-DMT) used in a naturalistic group setting is associated with unintended improvements in depression and anxiety.” The American journal of drug and alcohol abuse 45.2 (2019): 161–169.
Connect with us on social media:

@Dr_Alex_Crimi

@dr.alecrimi
