Building an Effective Self-Service Data Platform: Key Insights
Written on
Understanding Self-Service Data Platforms
Consider the experience of shopping at a grocery store: you grab a basket, select your items, and checkout without any human interaction. Now, imagine your business stakeholders addressing specific questions by bringing their data into a data lake or warehouse, refining it, and generating business intelligence reports—all independently of a dedicated team. This is the essence of a self-service data platform: "A system that empowers stakeholders to ingest, curate, share, and report on their own data without relying on others."
Traditionally, centralized teams have overseen data platforms, controlling both infrastructure and data management. This often leads to bottlenecks in new initiatives. In response, many organizations have embraced distributed data ownership—or multitenancy. In this model, each data owner is responsible for managing their own data pipelines, while the platform team focuses solely on the infrastructure and enhancing its capabilities. Although empowering users to be self-sufficient is an attractive concept, there are significant challenges to address.
The Top Four Challenges of Self-Service Data Platforms
While the underlying technology is crucial, most challenges stem from cultural and operational factors. Here are the key obstacles:
1. Securing Executive Support
Transitioning to a self-service data platform requires a significant change in mindset rather than just technology. Gaining support from senior management is vital for the success of this initiative, as their endorsement guides the organization in effectively utilizing data.
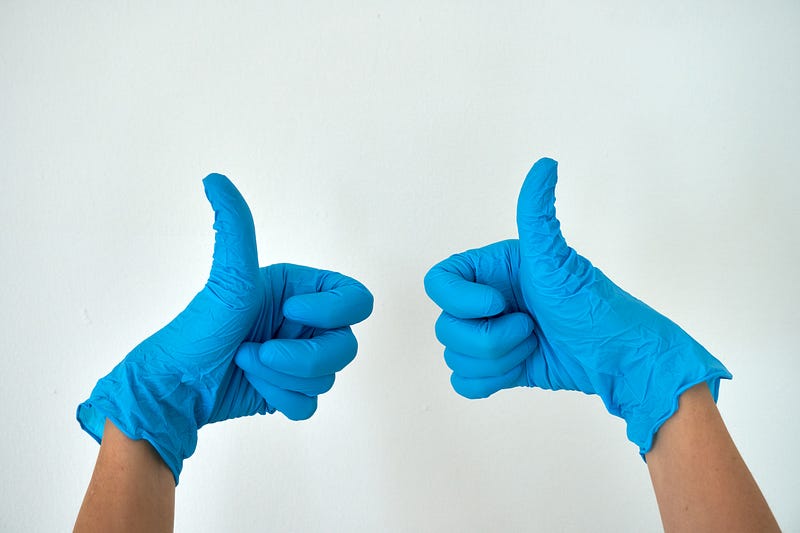
Leadership plays a critical role in promoting the idea of self-service analytics. If executives don’t recognize its value, they will struggle to persuade their teams to adopt it.
2. Skills Gap
A common concern among data owners is the lack of skilled data engineers within their teams. The foundation of self-service relies on empowering users, often analysts or business users without engineering backgrounds.
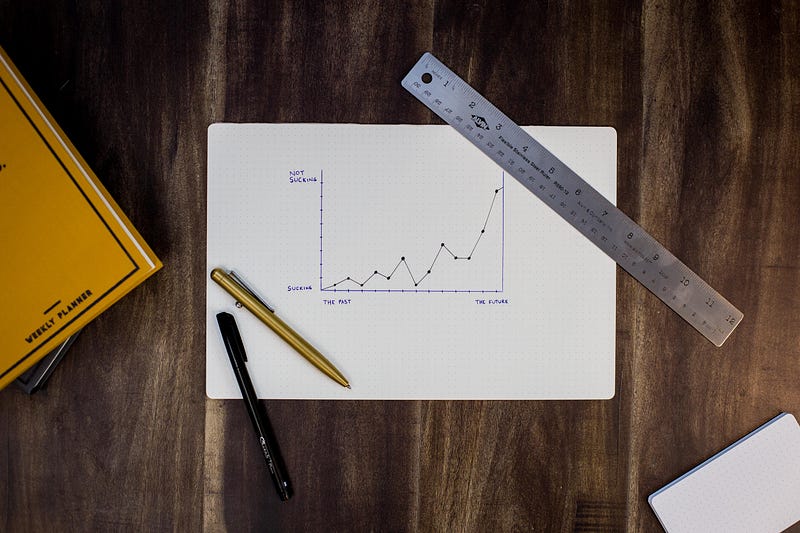
To bridge this gap, organizations may need to hire data engineers, which can be a lengthy and costly process, particularly when multiple teams are involved.
3. Repetitive Solutions
Each team has its own working methods based on priorities and objectives, leading to diverse practices in data engineering. This lack of standardization can hinder the efficiency of data sharing and collaboration.
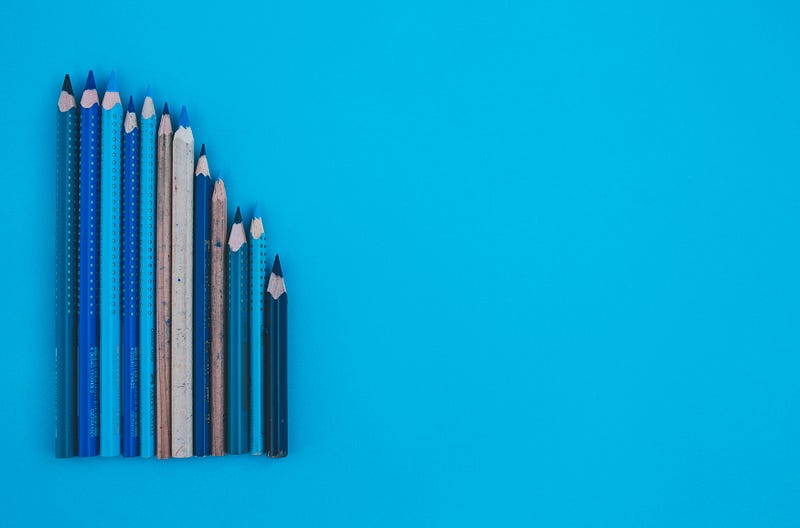
When teams independently tackle similar problems, it often results in redundant efforts and inefficiencies.
4. Access Management Challenges
Delegating control of data and pipelines to various business teams introduces security and access issues. Increased access can lead to data proliferation and the risk of leaks.
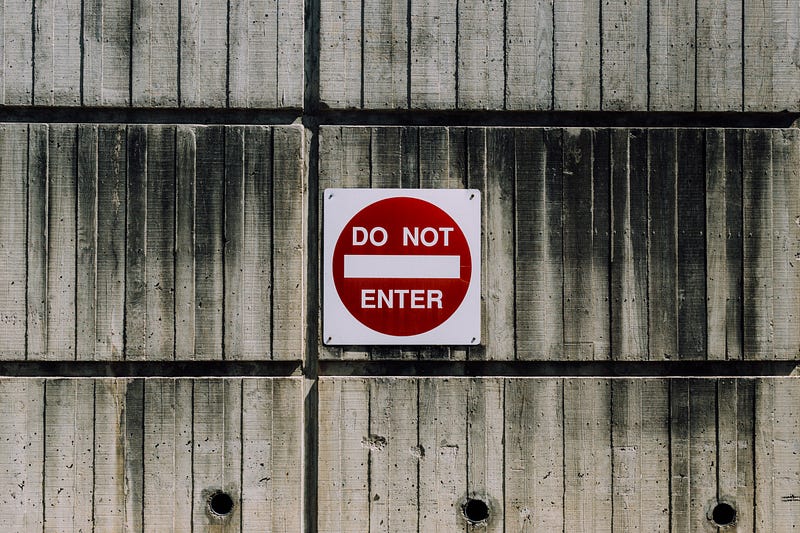
Striking the right balance between user access and security is essential to mitigate operational risks while enabling analytics.
Strategies for Overcoming Challenges
#### Engage Stakeholders
Promoting your data platform concept effectively requires engaging advocates. Presenting the benefits and capabilities of the platform before its launch can create enthusiasm and support.
#### Initiate Small-Scale Self-Service
Instead of attempting to fully empower teams immediately, consider starting with self-service reporting. Gradually introduce responsibilities to prevent overwhelming users while establishing a foundation for future growth.
#### Implement Standard Practices
To avoid chaos, set clear standards and train engineering teams to adhere to them. This ensures consistency in data engineering processes.
#### Foster Collaboration
Encouraging continuous collaboration among distributed teams is crucial. Regular training, discussions, and workshops can help share insights and best practices, boosting acceptance of the platform.
Conclusion
A data platform is now a fundamental aspect of business strategy, with organizations eager for rapid insights. Careful planning and execution are vital to ensure that the platform meets user needs efficiently. Regardless of its design, prioritize stakeholder usage to ensure its success.
"Build a platform—prepare for the unexpected... you’ll know you’re successful when the platform you’ve built serves you in unexpected ways." — Pierre Omidyar
Chapter 2: Practical Insights from Real-World Examples
To deepen your understanding of self-service data platforms, check out these insightful videos:
The first video, "Self-Serve Data Infrastructure by Example," provides real-world scenarios and strategies for implementing self-service data solutions.
The second video, "How to Build a Self Serve Data Platform? - Denise Schlesinger," offers expert guidance on developing an effective self-service data environment.